Table of Contents
Agentic AI is a form of artificial intelligence that can make decisions and take action independently to achieve specific objectives, all without direct human intervention. They are designed in such a way that they are continuously learning from these decisions, actions, and their results. These systems are designed to perceive their environment, to reason, to plan, and to adapt to new information, which enables them to operate autonomously within the defined parameters.
In this article we will explore the main components of Agentic AI and how they all work together to do the job. We will also look at some popular use cases of Agentic AI and ethical considerations that come with it so you can use it mindfully.
How does Agentic AI Work?
Agentic AI enables systems to operate with a high degree of autonomy, adaptability, and decision-making capability. Let’s understand how it works and explore key components and processes that allow it to function effectively.
Key Components
- Perception: Agentic AI systems continuously collect data from their environment through sensors, user inputs, or data streams. These form the basis for understanding and interacting with the world.
- Cognition: Advanced algorithms, including machine learning and deep learning, are employed by the system to process and interpret the collected data. These cognitive processes allow the AI to understand advanced patterns in data, make inferences, and predict outcomes.
- Decision-Making: Through cognitive insights, Agentic AI weighs possible actions and selects the best course toward realizing its goals. This process often involves probabilistic reasoning, weighing potential benefits against risks.
- Action: After making decisions, AI takes actions within its environment. Actions can include physical motion in robotics, responses in conversational agents, or initiating processes in software applications.
- Learning and Adaptation: Agentic AI systems apply learning mechanisms to develop behaviors through experience and feedback, such as reinforcement learning. This continuous learning loop enables the AI to improve over time and adapt to new situations.
So how does it all work together?
Agentic AI works through a structured workflow that starts with data acquisition, where the system collects real-time information from diverse sources to stay updated about its environment. Once the data is gathered, processing begins, using advanced computational models to analyze the information, extract insights, identify patterns, and understand the context. With this processed information, the AI sets goals, articulating specific objectives aligned with its programming and insights.
Next, the system creates a strategic plan, considering multiple steps and contingencies to achieve its goals effectively. After planning, the AI enters an execution phase, where it interacts with its environment or users to perform tasks. Finally, feedback is integrated into the system, allowing it to monitor outcomes, refine behavior, and make better decisions in future interactions. This cycle of acquisition, analysis, action, and refinement enables Agentic AI to function autonomously in dynamic scenarios.
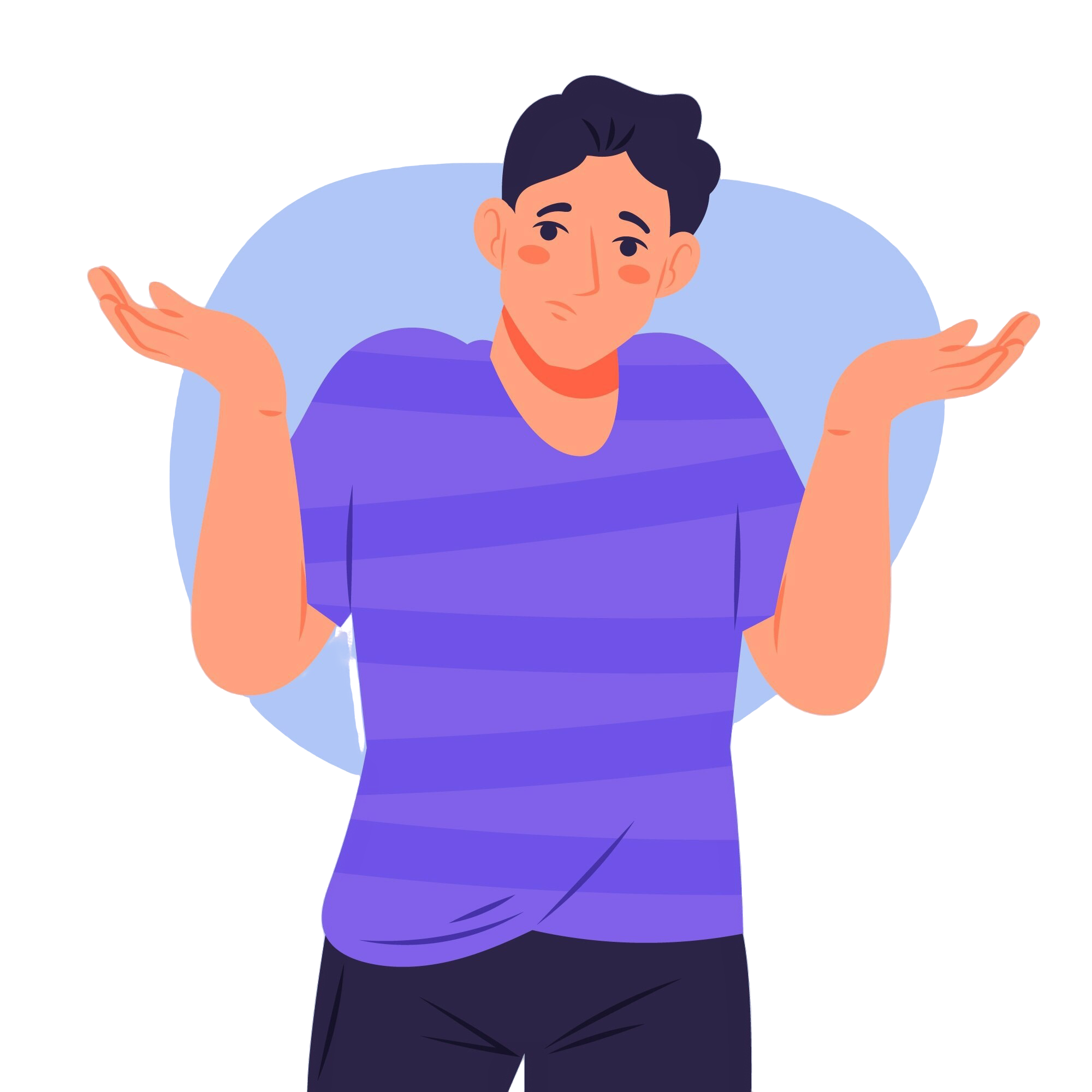
There are a lot of terms and concepts going around in the world of AI. It’s very easy to be confused by all these terms, so let’s quickly discuss some (that might potentially cause confusion) and compare them with our focus for today—Agentic AI.
Agentic AI vs. AI Agent
At their core, AI agents are computational systems designed to interact with their environment through sensors and actuators. The building blocks of AI agents are nothing but Large Language Models(LLMs), they produce their responses based on the data used to train them and are bounded by knowledge and reasoning limitations. Just as humans use their senses to gather information and their bodies to take action, AI agents employ various mechanisms to perceive and influence their surroundings. These agents operate on a continuous cycle of perception, reasoning, and action, known as the perception-action cycle.
To learn more about AI Agents, check out our comprehensive guide.
Aspect | AI Agents | Agentic AI |
---|---|---|
Autonomy | Operate within predefined rules; limited decision-making capabilities. | Exhibit high autonomy; capable of independent decision-making and goal-setting. |
Learning Ability | Generally lack learning capabilities; require manual updates for new tasks. | Possess learning mechanisms; adapt and improve performance over time through experience. |
Task Complexity | Suited for specific, well-defined tasks (e.g., scheduling, information retrieval). | Designed to handle complex, unstructured tasks in dynamic environments (e.g., autonomous driving). |
Adaptability | Limited adaptability; function within the scope of their initial programming. | Highly adaptable; can adjust to new, unforeseen situations without human intervention. |
Proactivity | Reactive; respond to specific inputs or triggers. | Proactive; can anticipate needs and take initiative without explicit prompts. |
Agentic AI vs. Generative AI
Generative AI creates new content from input data, such as text, images, or music. For example, GPT-4 generates human-like text, while GANs create realistic images. Generative AI is primarily reactive, responding to user inputs.
To learn more about Generative AI, check out this comprehensive guide.
Aspect | Generative AI | Agentic AI |
---|---|---|
Primary Function | Create new content based on input data | Perform tasks autonomously to achieve goals |
Interactivity | Reactive; responds to user inputs | Proactive; initiates actions without human input |
Complexity | Generates content like text, images, or music | Manages complex tasks and decision-making |
Adaptability | Limited to the scope of training data | Learns and adapts to new situations |
Examples | Text generation, image creation, music composition | Autonomous customer service agents, robotic process automation |
Applications of Agentic AI
Agentic AI is changing a lot of industries by enhancing efficiency, decision-making, and the ability of different systems to function independently without needing human intervention. Let’s look at some examples of how it’s doing that.
Agentic AI can allow for personalized support in customer service by automating responses and resolving customer issues. They can handle routine inquiries, which can allow the “human” team members to focus on more complex problems, which can eventually help improve response times and customer satisfaction. For example, an AI-driven chatbot can provide instant answers to frequently asked questions, or a more advanced system can also be used to analyze customer sentiment to tailor interactions appropriately.
Business operations can also be streamlined using Agentic AI to enhance the decision-making process, which can ultimately help boost the overall efficiency of the enterprise. AI-driven workflows can automate any repetitive tasks, manage data analysis, and generate insights, freeing employees to concentrate on strategic initiatives. This integration leads to more agile and responsive business operations since it also has the capability to quickly adapt to changing data and market conditions.
Agentic AI systems function as autonomous agents across various domains, including robotics, gaming, and healthcare, performing tasks without human oversight. In robotics, AI agents can navigate and interact with environments independently. In gaming, they can control non-player characters to create more dynamic experiences. In healthcare, autonomous AI can monitor patient vitals and administer treatments, enhancing patient care and operational efficiency.
Ethical Considerations
If you plan on using agentic AI, it is essential to ensure that there is a robust framework in place to govern the whole system to ensure ethical decision-making, transparency, and accountability. It’s also really important to establish clear guidelines and oversight mechanisms to mitigate any potential risks associated with agentic AI systems.
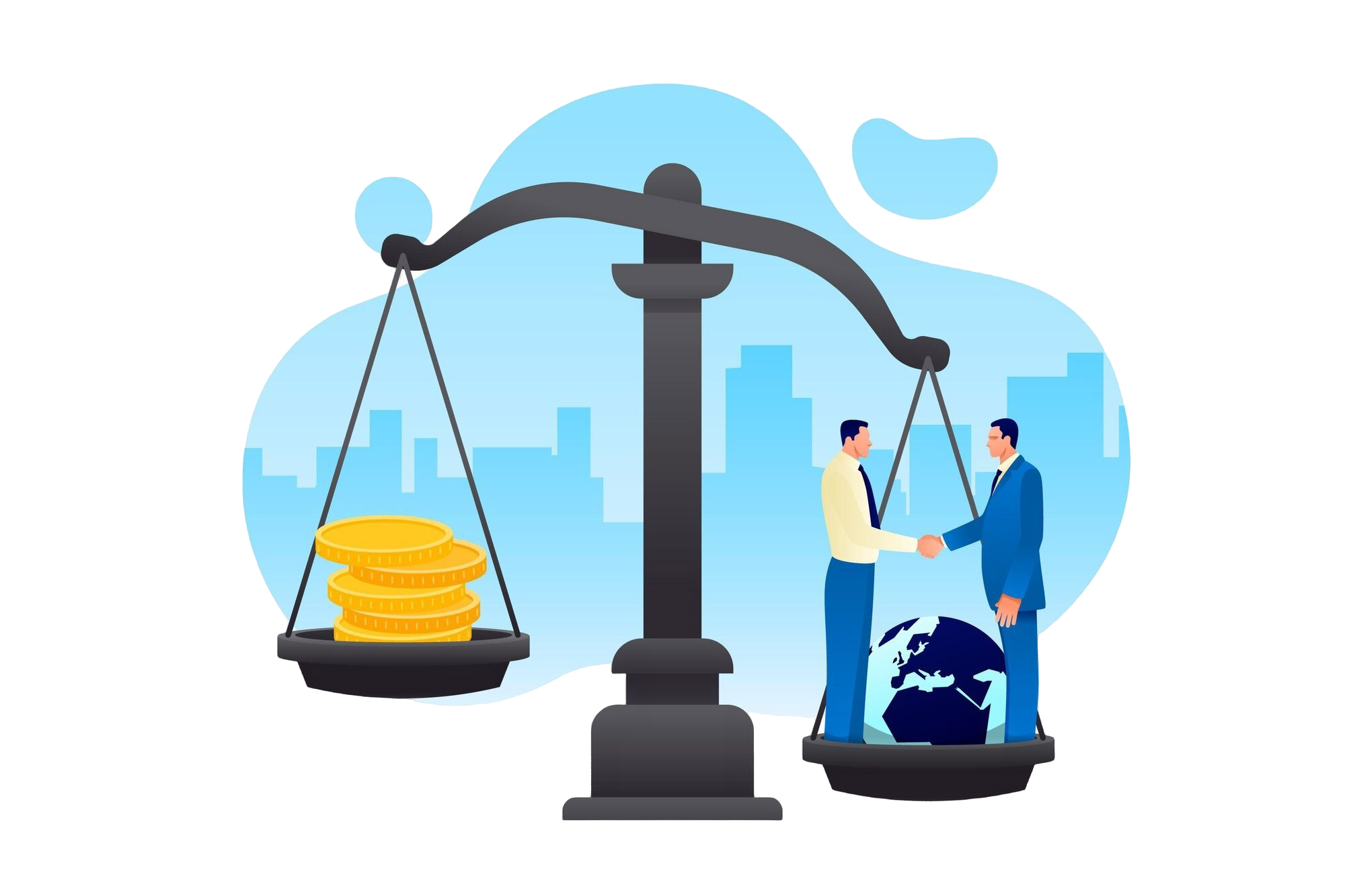
- Ethical Decision-Making: Agentic AI systems operate autonomously, making decisions that can significantly impact individuals and society. Ensuring these decisions align with ethical standards requires the implementation of comprehensive ethical guidelines. This includes addressing issues such as bias, fairness, and respect for user autonomy. For instance, AI agents should be designed to avoid discriminatory practices and to respect user privacy.
- Transparency: Transparency in agentic AI involves making the decision-making processes of AI systems understandable to users and stakeholders. This openness helps build trust and allows for informed oversight. Transparent AI systems disclose their AI nature, provide explanations for their actions, and make their underlying data and algorithms accessible for scrutiny. Such transparency is crucial in applications where AI agents participate in deliberative processes, ensuring that their contributions are appropriately understood and evaluated.
- Accountability: Establishing accountability mechanisms ensures that when agentic AI systems cause harm or make errors, there are clear protocols for addressing these issues. This includes defining responsibility for the actions of AI agents, whether it lies with developers, deployers, or other stakeholders. Accountability frameworks are essential to provide recourse for affected parties and to maintain public trust in AI technologies.
- Governance Frameworks: Developing governance frameworks for agentic AI involves creating policies and regulations that guide the ethical development and deployment of AI systems. These frameworks should be comprehensive, covering aspects from the design phase through to deployment and ongoing operation. They must also be adaptable to keep pace with the rapid evolution of AI technologies. Implementing such frameworks helps ensure that AI innovations remain equitable and aligned with societal values.
- Oversight Mechanisms: Effective oversight mechanisms involve continuous monitoring and evaluation of agentic AI systems to ensure compliance with established ethical guidelines and governance frameworks. This can include regular audits, impact assessments, and the involvement of independent review boards. Such mechanisms are vital to detect and address issues promptly, preventing potential harm and ensuring that AI systems operate as intended.
Future of Agentic AI
Agentic AI applications (like the ones we just discussed) are only just the beginning of a coming wave of change, even though they have already started to significantly alter work processes. You can expect leading companies to go through significant transformations over the next five years, which include incorporating AI and automation into every aspect of their business operations. This will give these businesses the ability to function with previously unheard-of speed, agility, and efficiency and enable them to distinguish themselves through outstanding client interaction and dynamic response to upcoming obstacles.
Agentic AI integration will increase engagement, redefine employee roles, and increase productivity. AI essentially enables workers to concentrate on higher-value work by automating repetitive and routine tasks, which promotes creativity and job satisfaction. The goal of this evolution is to enable organizations to prosper in a world where human-machine collaboration is the norm, not just to increase efficiency. So it is safe to say that Agentic AI has a bright future ahead of it. As technology advances, it will continue to transform workflows, change industries, and transform how people interact with machines.
FAQs
What is the meaning of agentic AI?
What is the difference between Agentic and Non-Agentic AI?
Agentic AI systems are capable of autonomous decision-making and action-taking to achieve specific goals. Non-agentic AI systems, on the other hand, operate based on predefined rules or inputs and lack the ability to make independent decisions or pursue goals without human intervention.
How is Agentic AI different from Robotic Process Automation (RPA)?
What is the difference between Generative AI and Agentic AI?
Generative AI focuses on creating new content, such as text, images, or music, based on the data it has been trained on. In contrast, Agentic AI is designed to autonomously pursue complex goals and workflows with limited direct human supervision.